Building Health Guardrails
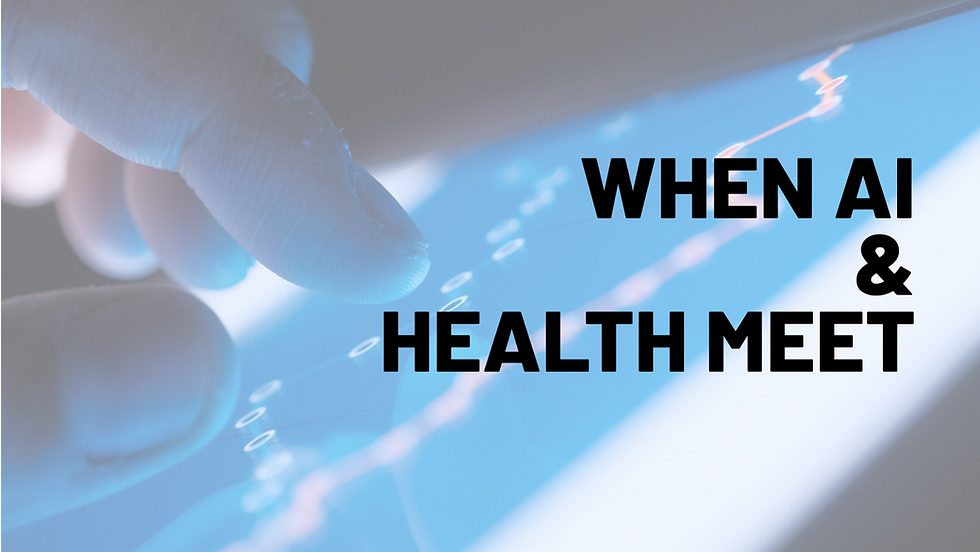
AI can help when we have issues with geographical access or an inadequate number of health providers (thanks @Ricardo, who spoke about this at the @EuroDig yesterday). It can help in crunching numbers and reduce the time for experimentation in trials BEFORE it reaches human trials. Think of AI identifying molecules (like AlphaFold), or it can be used in CRISPR technologies for DNA therapies (it can make mistakes) and editing. It can help in assisting in diagnosis (accuracy rates are 75-95% - or error rates are 25-5%).
But can it be misused? @MorningBrew reported the case of DoneGlobal, a firm charged with helping prescription misuse of ADHD pills. They cite this interesting article from @Vox, which found that thanks to social media, people were self-diagnosing themselves using social media. The problem got exacerbated with telehealth (we need human-in-the-loop). We see such issues with mental health chatbots, robotic surgery, patient scores, etc. Of course, these technologies need feedback from humans to make them better and more safeguards if involved with people.
How can we build guardrails? This topic is something I have been talking about for some time, and it fits in with the work I am doing with @TheDigitalEconomist in the Applied AI Group in the Centre of Excellence on Human-Centered Global Economy.
1. A shortage of human talent does not mean AI is the permanent answer – it can be a temporary fix, but in the long term, you need to train more people – people have empathy and look beyond the answers an AI may pick up. It may mean changing regulations to have more practitioners (we legally introduced back midwives in many countries when there was a shortage of obstetricians or recruited “nurse” volunteers during times of war).
2. AI can definitely augment human decision-making. Still, the concerned human – the doctor, radiologist, researcher, pharmacist, policy maker, etc.- must understand HOW AI works to know when to trust its outputs (NOTE: I did not say judgment; it is an algorithm and not human).
3. Human-centeredness – when profit is put before people, ethical lapses will often occur. Also, the idea of RCTs (versus synthetic data) is that this people-centric data is vital for the long-term safety of the human—the qualitative data matters.
4. Human-in-the-Loop – build systems for human intervention and reflection in decision-making. The law penalizes HUMANS over AI and AI companies (and big tech companies have more money for legal lawsuits). Hence, we need to give Humans every opportunity to reflect on the decisions they take on others. Especially if the risk of the decision's impact on the human increases (this is a perception).
5. Include health sandboxes. This is important before deploying. The issue is that many technologies are moving from adjacent industries – Electronic Health Records being used for identifying health markers, Wearables into the health category, Ear Hearing devices (like iPods/VR headsets), and brain scans….are we prepared?
Comments